This insight examines a recently published Report on Generative Artificial Intelligence Patents by the World Intellectual Property Organisation, as of mid-2024.
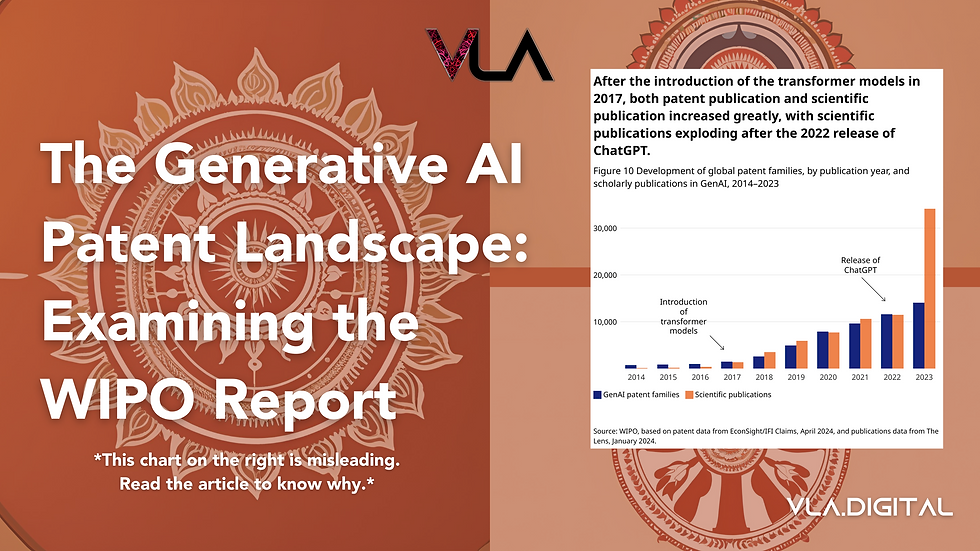
Now, let's address a caveat before delving into the analysis and the WIPO report itself.
It's important to note that this report may not serve as the definitive authority on AI patentability within the WIPO's international intellectual property law framework.
While the report provides valuable insights, certain sections discussing the substantive features of Generative AI and related aspects might not directly reflect WIPO's official stance on AI patentability.
This caveat is crucial for two reasons:
Evolving Landscape: The AI patentability landscape is still developing, and individual countries are establishing their own legal frameworks, positions, and case law on the subject.
International Framework: The creation of an international intellectual property law framework under WIPO for AI patentability remains uncertain, as aspects related to economic-legal contractual rights and knowledge management may evolve.
The Three Perspectives of Analysis in this Report
This report is based on three key aspects of analysis, or let us say, three perspectives of analysis, when it examines the Generative AI Landscape:
– The first perspective covers the GenAI models. Patent filings related to GenAI are analyzed and assigned to different types of GenAI models (autoregressive models, diffusion models, generative adversarial networks (GAN), large language models (LLMs), variational autoencoders (VAE) and other GenAI models).
– The second perspective shows the different modes of GenAI. The term “mode” describes the type or mode of input used and the type of output produced by these GenAI models. Based on keywords in the patent titles and abstracts, all patents are assigned to the corresponding modes: image/video, text, speech/voice/music, 3D image models, molecules/genes/ proteins, software/code and other modes. Introduction
– The third perspective analyzes the different applications for modern GenAI technologies. The real-world applications are numerous, ranging from agriculture to life sciences to transportation and many more.
Here's an example to illustrate each perspective:
GenAI Models Perspective
Let's consider a hypothetical company, AICorp, that has filed a patent for a new generative adversarial network (GAN) architecture. The patent describes improvements to the generator and discriminator components of the GAN, enabling it to produce higher quality synthetic images. In the WIPO report's analysis, this patent would be assigned to the "generative adversarial networks (GAN)" category under the GenAI models perspective.
GenAI Modes Perspective
Now, suppose AICorp's patented GAN model is specifically designed to generate realistic images and videos. The patent abstract and title mention "high-resolution image synthesis" and "video generation."Based on these keywords, the WIPO report would classify this patent under the "image/video" mode in the second perspective, which looks at the types of input and output data the GenAI model handles.
GenAI Applications Perspective
Finally, let's say AICorp's patent describes potential applications of their GAN model in creating virtual product demos for e-commerce websites and generating synthetic data for autonomous vehicle perception systems. In the third perspective, the WIPO report would categorize this patent under both the "business solutions" and "transportation" application areas, based on the real-world use cases mentioned.
What is GenAI then?
Interestingly, this report takes note of how Generative AI is defined in the European Union's Artificial Intelligence Act. Here is an interesting excerpt from the "Background and Historical Origins" segment:
From the point of view of general users, one key aspect is that unlike the traditional "supervised" machine learning models, which require a large amount of task-specific annotated training data, these models can generate new content just by writing natural language prompts. Therefore, using GenAI tools based on these models does not require technical skills. For the first time, modern cutting-edge AI becomes directly accessible to the general public.
The report also recognises the role of synthetic content in shaping the effectiveness of Generative AI deliverables. Here is how the term 'synthetic content' is defined:
Synthetic data is annotated information that computer simulations or algorithms generate as an alternative to real-world data. It usually seeks to reproduce the characteristics and properties of existing data or to produce data based on existing knowledge (Deng 2023). It can take the form of all different types of real-world data. For example, synthetic data can be used to generate realistic images of objects or scenes to train autonomous vehicles. This helps for tasks like object detection and image classification. Because of synthetic data, millions of diverse scenarios can be created and tested quickly, overcoming limitations of physical testing.
Methodology for patent analysis
Now, the Appendix A of the Report acknowledges that GenAI itself is a modern concept without any clear definition. In that regard, even the patent classes of Generative AI are not fully established yet.
For example, the Cooperative Classification Scheme's (CPC) G06N3/045 ("Auto-encoder networks; Encoder-decoder networks") is the most relevant patent classification for GenAI, which is a sub-group of G06N3/02 Neural Networks. Many of the sub-groups in this area were recently introduced in 2023 and are currently being reclassified.
As per the Report, there is no specific class dedicated to GenAI. However, GenAI can be considered a broad concept encompassing the application of various software methods to vast datasets ("modes"), addressing multiple applications. This is evident in the use of numerous generic terms in modern patents, often without defining the underlying technology used, which is assumed to be known by skilled practitioners in the field.
Here is an excerpt from Appendix A explaining how they had developed a specific approach for capturing patents involving 'Generative AI'.
We had to develop a specific approach for capturing patents, involving the generation of digital entities, such as images, text or data through the use of specific machine learning algorithms. To achieve this, we used a two-stage approach: first, we combined classical patent searches together with prompts using our AI tool (see Appendices A.4 and A.5 for the patent searches and prompts) to retrieve a first patent dataset with high recall. Second, we refined the previous set using a trained BERT classifier to increase precision. This approach helps to avoid patents that are not “GenAI” according to the usually accepted definition, but that might generate products or other things (such as 3D printers or cameras) by using AI techniques somewhere in a process.
Here's also the methods of initiating patent searches:
Method 1a: A patent search using generic terms such as “generative AI” to locate patents using specific keyword concepts only. These concepts are searched more broadly or narrowly in different patent classifications.
Method 1b: Five patent searches for the specific search concepts: Generative Adversarial Networks (GAN), Autoregressive Models, Diffusion Models, LLMs and Variational Autoencoders. These five concepts are considered as almost a synonym to the concept “generative AI.”
Method 2: About one hundred prompts for various concepts of GenAI and its use, by using EconSight’s advanced AI search algorithms.
Since these aspects have been addressed, let us now examine the contents of the report, for gaining clarity.
Patent Trends in GenAI Models
Patentability Economics Landscape
China's dominance in all five core GenAI models (diffusion models, autoregressive models, GANs, VAEs, LLMs) in terms of patent families has significant implications for the global AI innovation landscape and the economics of patentability.
Diffusion Models: China's lead is most pronounced in diffusion models, with 14 times more patent families than the US since 2014. This suggests Chinese inventors and companies are aggressively seeking to protect their intellectual property in this emerging GenAI technique, which could give them a competitive edge in commercializing related products and services. The high volume of Chinese patents may create barriers for other countries' companies to enter this space without risking infringement.
Autoregressive Models: China also has a very high global share of patents in autoregressive models. As these models are key to applications like text generation, China's strong IP position could enable its companies to capture value in emerging markets for GenAI-powered content creation tools and services.
GANs: South Korea and India have a relatively high proportion of their GenAI patents in GANs, a widely used technique for generating synthetic images and videos. This focus may align with national industrial strategies around digital content production. However, they may face challenges in global markets given China's overall lead in GAN patents.
VAEs and LLMs: The US has strengths in VAEs and LLMs relative to its overall GenAI patent position. This could stem from fundamental research breakthroughs by American universities and companies. Protecting these innovations through patents can help the US capture economic value, but Chinese firms may be able to develop workarounds or alternatives.
Japan: The lack of categorization of Japanese GenAI patents suggests they may be taking a more exploratory approach, patenting novel architectures beyond the mainstream models. While riskier, this strategy could pay off if they develop superior techniques that become globally adopted standards.
AI and Law Perspective
The stark disparities in GenAI patenting across countries and models raise important legal and policy questions:
Patent Quality: The high volume of Chinese GenAI patent filings, especially in diffusion models, raises potential concerns around patent quality and validity. Less rigorous examination standards could lead to overbroad or dubious patents being granted, fueling uncertainty and litigation.
Disclosure: GenAI models rely heavily on training data and algorithms. Current patent laws requiring disclosure of inventions may not be adequate for AI innovations, where details of datasets and model architectures are key. Lack of transparency could hinder follow-on innovation.
Infringement: The complexity of GenAI models makes it difficult to assess infringement. Overly broad patent claims could stifle innovation and competition if companies fear lawsuits. Skilled legal and technical analysis will be needed to determine the scope of protection.
Exceptions and Limitations: Countries may consider enacting targeted exceptions and limitations to patent rights for GenAI in order to enable research, interoperability and downstream use. But these need to be carefully crafted to avoid undermining incentives for innovation.
Patent Trends in GenAI Modes
Patentability Economics Perspective
The dominance of China across all GenAI modes in terms of patent families over the last decade has significant economic implications for the global AI innovation landscape.
Image/Video GenAI: China's strong focus on image/video-based GenAI, with nearly 13,000 patent families since 2014, positions it to capture significant value in the growing market for AI-generated visual content. This could give Chinese companies a competitive edge in fields like entertainment, gaming, and design.
Text and Speech/Voice/Music GenAI: China's high volume of patents in text and speech/voice/music GenAI, key data types for large language models (LLMs), suggests it is well-positioned to monetize AI applications in content creation, virtual assistants, and customer service.
Molecules/Genes/Proteins GenAI: The high growth rate of Chinese patents in this area (64% annually from 2021-2023) indicates a strategic focus on applying GenAI in the lucrative biotech and pharmaceutical industries. Securing broad patent protection could enable Chinese firms to extract significant licensing revenues.
US Strengths
The US has a relatively high global share of patents in software/code and molecules/genes/proteins GenAI. Focusing patenting activity in these complex, high-value areas could help US companies maintain competitiveness and pricing power.
Japan and South Korea
The emphasis on speech/voice/music GenAI in these countries aligns with their strengths in consumer electronics and suggests potential for capturing value in the growing smart device and IoT markets. However, Japan's recent decline in patenting activity may put it at a disadvantage.
AI and Law Perspective
The dominance of China across all GenAI modes in terms of patent families has significant implications that need to be considered in light of differing national legal frameworks around intellectual property and artificial intelligence. From a comparative law standpoint, China's patent system has some notable differences from the US and European systems that could impact GenAI inventorship:
China has a "first-to-file" system that grants patents to the first party to file an application, regardless of the date of invention. The US recently switched to a first-inventor-to-file system but still provides limited grace periods for inventors' own disclosures. This could incentivize a rush to file GenAI patents in China.
China allows a broader scope of patentable subject matter, including software, business methods and AI algorithms. The US and EU have more restrictions on such patents. Chinese GenAI inventors may face fewer eligibility hurdles.
China has requirements for foreign entities to file patents through a licensed Chinese patent agent and undergo a security review for inventions made in China. This could advantage domestic Chinese GenAI inventors over foreign ones.
In terms of AI-specific regulations, China was one of the first to issue dedicated rules on generative AI services in 2023. The Interim Measures require providers to respect IP rights, avoid illegal content, and undergo security assessments - but overall take a relatively permissive approach focused on promoting the GenAI industry.
By contrast, the draft EU AI Act proposes a more restrictive, risk-based approach requiring conformity assessments and prohibiting certain high-risk applications. The US has issued guidance like the AI Bill of Rights but not yet binding AI regulations at the federal level.These regulatory divergences, combined with China's first-to-file system and broader patent eligibility, could further reinforce its leading position in GenAI patenting. Chinese inventors may face lower regulatory barriers and have greater incentives to seek patents.
However, concerns around explainability, transparency and potential bias in GenAI systems may pose challenges under existing legal principles in multiple jurisdictions:
AI inventorship remains a grey area, with the US, EU and UK patent offices denying applications listing AI systems as inventors. Only South Africa and Australia have allowed it so far. Attributing GenAI inventions could prove legally tricky.
Product liability and tort law principles around foreseeability, control and disclosure duties could be strained by opaque GenAI systems making autonomous decisions. Apportioning liability between GenAI developers, deployers and users is an open question.
Data privacy laws like the EU's GDPR and China's PIPL impose restrictions on the use of personal data to train AI that could impact GenAI development. Differing national standards complicate compliance.
Connection between GenAI models and GenAI modes
The patent analysis reveals a strong interdependence between specific GenAI models and the types of data they process, which has significant implications for the patentability and legal protection of GenAI innovations.
Text and Large Language Models (LLMs)
The finding that text is the most commonly used data type for LLMs has important economic and legal ramifications:
LLMs trained on vast text corpora may raise copyright and fair use issues, as the training data likely includes copyrighted works. The extent to which such use constitutes infringement or is protected under fair use doctrines remains a grey area.
The generated text outputs of LLMs could potentially infringe on the intellectual property rights of the original text used in training. Establishing clear guidelines for attributing authorship and ownership of LLM-generated content is crucial.
Patenting LLM innovations requires careful consideration of the model's novelty and non-obviousness, as well as the sufficiency of disclosure, given the opacity of the training process.
Speech/Voice/Music and GANs/VAEs
The importance of speech, voice, and music data for GAN and VAE models presents its own set of legal challenges:
Using copyrighted audio data for training GANs and VAEs without permission may constitute infringement. Developers must ensure they have the necessary licenses or rely on public domain or freely licensed datasets.
The generation of synthetic speech or music that closely mimics real individuals or artists could give rise to privacy, publicity rights, and trademark issues. Clear guidelines are needed to balance innovation with protecting individual rights.
Patenting speech/voice/music-related GANs and VAEs requires careful drafting to capture the key innovative aspects while avoiding overly broad claims that may be invalid or difficult to enforce.
Image/Video and GANs
The dominance of GANs in processing image, video, 3D model, and software/code data has significant implications:
Training GANs on copyrighted images or videos without authorization may infringe on the rights of content owners. Developing robust licensing frameworks and fair use guidelines is essential.
The generation of synthetic media that is indistinguishable from real content raises concerns about deepfakes, misinformation, and fraud. Legal frameworks must adapt to address these risks while enabling legitimate applications.
Patenting GAN innovations requires a nuanced understanding of the model architecture, training process, and applications to craft claims that are both novel and adequately described.
Molecules/Genes/Proteins and GANs/VAEs
The use of GANs and VAEs for processing molecular, genetic, and protein data presents unique legal considerations:
The generation of novel molecules or proteins using AI may challenge traditional notions of inventorship and patentability. Clarifying the eligibility of AI-generated innovations is crucial.
The use of proprietary or sensitive genetic data for training GANs and VAEs raises privacy and ethical concerns. Robust data governance frameworks and ethical guidelines are needed.
Patenting AI-generated molecules or proteins requires a delicate balance between incentivizing innovation and preventing overly broad monopolies that could stifle research.
Patent Trends in GenAI Applications
Patentability Economics Perspective
The strong interdependence between specific GenAI models and the types of data they process has significant economic implications for the patentability and commercialization of GenAI innovations.
Text and LLMs: The finding that text is the most commonly used data type for LLMs suggests a high potential for patenting text-based GenAI applications. As LLMs become more sophisticated in generating human-like text, there may be increased opportunities to secure broad patent protection for novel LLM architectures and training techniques. This could give patent holders a competitive edge in the growing market for AI-powered content creation, translation, and analysis tools.
Speech/Voice/Music and GANs/VAEs: The importance of speech, voice, and music data for GANs and VAEs highlights the potential for patenting innovations in AI-generated audio content. As these technologies advance, there may be valuable opportunities to patent novel GAN and VAE architectures optimized for generating realistic speech, music, and sound effects. Securing such patents could be particularly lucrative in the entertainment and gaming industries.
Image/Video and GANs: The dominance of GANs in processing image, video, 3D model, and software/code data suggests a rich space for patenting visually-focused GenAI techniques. Companies that develop novel GAN architectures or training methods enabling high-quality image, video, or 3D content generation may be able to secure valuable patents. These could be leveraged for licensing or to maintain a competitive advantage in fields like digital media, design, and visualization.
Molecules/Genes/Proteins and GANs/VAEs: The use of GANs and VAEs for processing molecular, genetic, and protein data indicates significant potential for patenting AI innovations in the biotech and pharmaceutical domains. Novel GAN or VAE techniques that can accurately generate or predict molecular structures, gene sequences, or protein foldings could be immensely valuable. Patenting such methods could help secure market exclusivity and attract investment in AI-powered drug discovery and precision medicine applications.
AI & Law Perspective
The interdependence between GenAI models and data types raises important legal considerations:
Apportionment of Rights in Collaborative GenAI Models
The finding that certain GenAI models like GANs and VAEs are particularly well-suited for processing specific data types like images, speech, and molecules highlights the need for clear legal frameworks governing the apportionment of rights in collaborative GenAI development.
As different organizations specialize in developing GenAI models optimized for specific data modes, complex questions arise around the ownership and licensing of the resulting IP when these models are combined or built upon.
Imagine a startup that develops a state-of-the-art GAN architecture for generating realistic images, which is then integrated by a larger company into a multimodal GenAI system that also processes speech and text using proprietary LLMs and VAEs. How should the IP rights and revenue streams from this combined system be allocated?
Collaborative GenAI model development will require adaptive licensing frameworks and revenue sharing models that equitably apportion rights based on the relative value contributed by each component. Smart contracts and blockchain-based systems for tracking model provenance and usage could help enable granular, automated allocation of IP rights.
Liability for Harmful Outputs from Multimodal GenAI
The use of different GenAI models for different data modes in a single system raises thorny questions around liability when that system produces harmful outputs:
A multimodal GenAI system that generates videos with synchronized speech and background music by combining the outputs of different sub-models for each mode (e.g. a GAN for video, LLM for speech, VAE for music) could produce defamatory content. But which model is liable - the one that generated the harmful speech, visuals, or both together?
As GenAI systems become more complex and incorporate an increasing variety of specialized models, a more nuanced approach to liability that considers each model's contribution to the final output will be needed. Blanket approaches that assign full liability to the overall system operator may not be suitable.
Legal frameworks must evolve to account for the modularity and composability of GenAI systems, perhaps by mandating embedded model-level monitoring and "explanation" capabilities that can help trace the provenance of harmful outputs to specific component models.
Need for Domain-Specific GenAI Governance Frameworks
The gravitational pull between certain GenAI model types and data modes points to the need for domain-specific governance frameworks tailored to the unique risk profiles of each pairing:
The use of GANs for generating highly realistic images and videos requires governance frameworks focused on mitigating risks like deepfakes, disinformation, and infringement of image/likeness rights. Mandatory watermarking or "radioactive data" approaches may be needed.
LLMs used for high-stakes text generation tasks like medical or legal analysis may require specialized testing and certification regimes, as well as mandatory quality control measures like human-in-the-loop verification of outputs.
GenAI systems used for drug discovery and molecular design will need governance frameworks ensuring compliance with safety regulations, clinical trial standards, and IP protections around generated molecules.
Connection between core models and applications
The strong interdependence between specific GenAI models and application areas raises important legal considerations around intellectual property, liability, and the need for domain-specific governance frameworks:
Liability for AI-Generated Outputs in High-Stakes Domains
The dominance of certain GenAI models in high-stakes application areas like transportation (GANs), life and medical sciences (diffusion models), and banking/finance (autoregressive models) raises critical questions about liability for harmful or infringing outputs:
If a GAN-generated image used to train an autonomous vehicle leads to an accident, who is liable - the GAN developer, the vehicle manufacturer, or the fleet operator? Apportioning fault will require careful analysis of each party's role and the causal chain.
Diffusion models used to generate protein sequences for drug discovery may produce harmful molecular structures. Establishing liability will hinge on whether the harm was foreseeable and what safety checks were in place.
As GenAI models become more prominent in sectors with major public safety and socioeconomic ramifications, a clear liability framework tailored to the unique risks of each domain will be essential. This may require updates to existing product liability, negligence, and anti-discrimination laws.
IP Ownership of Outputs in Collaborative GenAI Applications
The use of different GenAI models for different applications in a larger system or workflow raises thorny questions around IP ownership of the ultimate output:
If a VAE used for anomaly detection in smart city sensor data feeds into a larger predictive maintenance system involving other GenAI and non-AI components, who owns the IP in the final insights - the VAE developer, the system integrator, or the city? Contracts will need to contemplate the "nesting" of GenAI within larger solutions.
LLMs used for creative text generation may be fine-tuned on an enterprise's proprietary data before being deployed in a customer-facing chatbot. Ownership of the generated text may be unclear between the LLM provider, the enterprise, and the end user. Terms of use and licensing agreements must directly address these scenarios.
As GenAI enables more "modular" and composable AI development, legal frameworks for IP allocation must evolve beyond the binary paradigm of human vs machine authorship. Nuanced approaches considering the relative contributions of different GenAI and human components will be needed.
Need for Domain-Specific GenAI Governance Frameworks
The clustering of certain GenAI model types around particular application domains highlights the need for tailored governance frameworks attuned to the unique technical and societal challenges of each domain:
The prominence of GANs in transportation applications like autonomous driving will require governance frameworks focused on ensuring verifiable safety, security, and interpretability of training data and outputs. Sector-specific standards and validation protocols may be needed.
The use of diffusion models for high-stakes life sciences applications like drug and protein design will require close coordination with health regulators to ensure compliance with safety and efficacy standards. Specialized approval pathways and monitoring mechanisms may be required.
LLMs powering legal and governmental applications will need robust governance to ensure adherence to due process, explainability, and non-discrimination principles enshrined in public law. Mechanisms for public participation and oversight in GenAI development and deployment will be critical.
In short, the strong convergence of certain GenAI models around high-stakes application areas necessitates a shift from one-size-fits-all AI governance to domain-specific approaches.
Limitations and future of patent analysis in relation to GenAI
The report rightly highlights the challenges traditional patent analysis methods face in keeping pace with the rapid evolution of digital technologies like GenAI. The use of pre-defined patent classification schemes and keyword searches can indeed lag behind the emergence of new concepts and terminology in these fast-moving fields.
However, the report could provide more concrete evidence to substantiate these claims. For instance, it would be helpful to see specific examples of GenAI-related patents that were missed or misclassified by conventional search methods. Quantitative data on the percentage of GenAI patents falling outside existing classifications, or the average time lag between a new concept emerging and it being added to patent taxonomies, would lend credibility to the argument.
The report cites the rapid user adoption of ChatGPT as an example of GenAI's accelerated development outpacing the patent system's response. While this anecdote is illustrative, it is somewhat misleading to conflate the speed of consumer adoption with the pace of technological change from a patentability perspective. The underlying machine learning techniques behind ChatGPT, like transformer architectures and large language models, have been the subject of patent filings for several years prior to the product's launch.
Similarly, the semantic ambiguity between "AI-generated" and "AI-assisted" content creation highlighted in the report, while valid, is not a new phenomenon unique to GenAI. Analogous challenges have long existed in software and business method patents, where the line between automation and human direction can be blurry. The report would benefit from acknowledging this continuity and explaining how GenAI may differ in the degree or implications of such ambiguity.
The potential of advanced AI tools like LLMs to enable more agile and intelligent patent search and classification, as alluded to in the report, is indeed exciting. However, the report seems to take an overly optimistic view of the current capabilities and readiness of these techniques. While promising, AI-based prior art search and patent landscaping are still nascent and face significant challenges around data quality, interpretability, and legal admissibility. For instance, the report mentions using "patent-trained LLMs" and "fine-tuned models" to collect relevant patents and map development trends. However, it does not address key questions such as:
How are these models trained and validated to ensure comprehensive and unbiased coverage?
How do they handle the "long-tail" of niche or emerging concepts that may be underrepresented in training data?
What mechanisms exist for human experts to scrutinize and contest the outputs of these "black-box" models?
The report would be strengthened by a more critical and concrete discussion of the current limitations and development roadmap for AI-based patent analysis.
Additionally, while the report understandably focuses on the benefits of AI for patent offices and applicants, it largely ignores the potential risks and challenges. These include issues around algorithmic bias and transparency, data privacy and security, and the need for significant upskilling of patent professionals. A more balanced and forward-looking analysis would consider these factors and highlight the need for proactive governance frameworks.
Conclusion
In conclusion, while the WIPO report identifies the disruptive potential of GenAI for patent analysis, its claims would be bolstered by more rigorous evidence, acknowledgement of historical continuities, and a critical examination of the current maturity and limitations of AI-based solutions. As GenAI reshapes the patent landscape, a nuanced and interdisciplinary approach that brings together technical, legal, and policy perspectives will be crucial to realizing its benefits while navigating its challenges.
The WIPO Report is still substandard in terms of its estimations, and in many sections of the report, too many colourable and misleading statements around Generative AI have been referred to, for example, on technical deliverables, the quantum of how patentability plays out in a clarified sense and others. The honest and reasonable aspect of this report is that WIPO and EconSight acknowledge the limitations in their report, especially on patent analysis, features of patentability, technical categorisation of Generative AI and the interplay of technical purpose & use cases of Generative AI.
This is why it is necessary to read the complete report beyond reading some stats believing numbers on how many GenAI patents exist in comparison to the number of scientific publications published. Many scientific publications, including those on Generative AI, have been found fake. However, most people do not care about nuances, and believe that hype cycles will fix problems. We hope this insight for Visual Legal Analytica has been helpful to all.
Thanks for reading this insight.
Since May 2024, we have launched some specialised practice-based technology law and artificial intelligence & law training programmes at indicpacific.com/train.
We offer special discounts for technology teams who may be interested in enrolling for the training programmes on bulk. Feel free to choose your training programme at indicpacific.com/train and contact us at vligta@indicpacific.com.
Comments