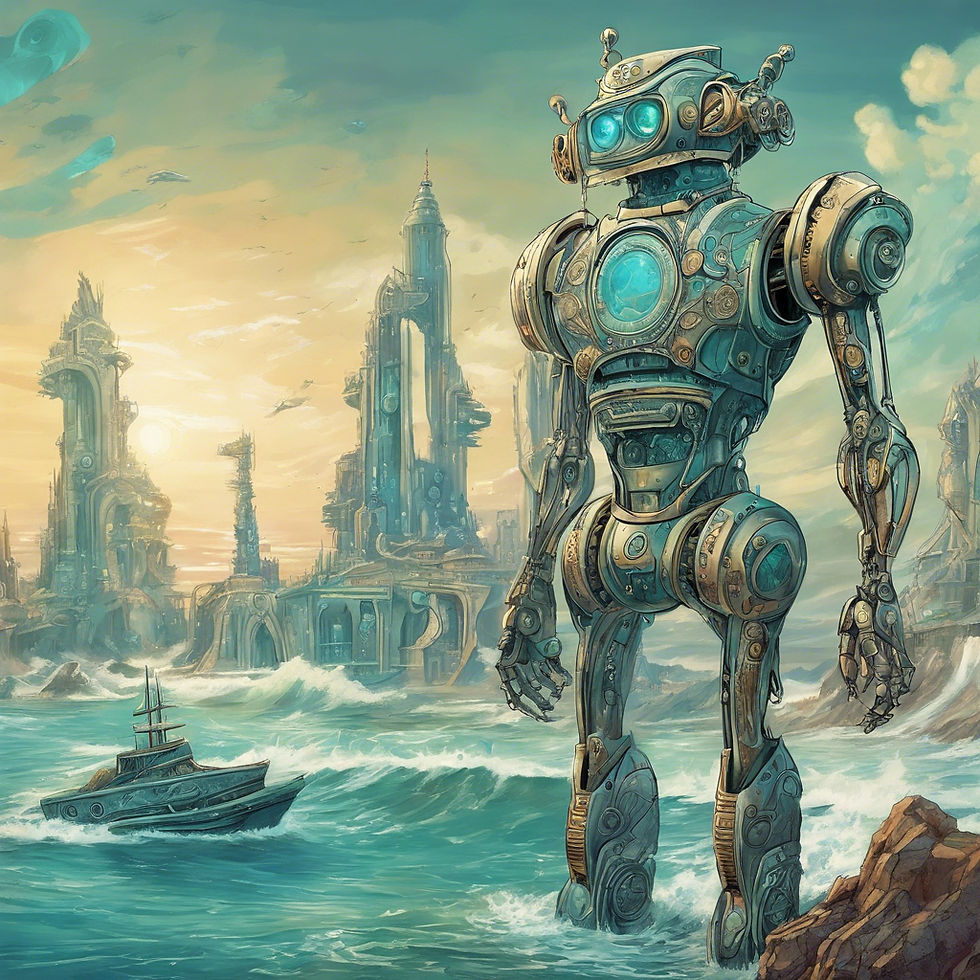
TESCREAL serves as a lens through which we can examine the motivations and potential implications of cutting-edge technological developments, particularly in the field of artificial intelligence (AI).
As these ideologies gain traction among tech leaders and innovators, they are increasingly shaping the trajectory of AI research and development. This insight brief explores the potential risks and challenges associated with the TESCREAL framework, focusing on anticompetitive concerns, the impact on skill estimation and workforce dynamics, and the need for sensitisation measures. By understanding these issues, we can better prepare for the societal and economic changes & risks that advanced & substandard AI technologies may bring.
It is crucial to consider not only the promises but also the pitfalls of the hype rapid advancement. This brief aims to provide a balanced perspective on the TESCREAL ideologies and their intersection with AI development, offering insights into proactive measures that can be taken before formal regulations are implemented.
Introduction to TESCREAL
The emergence of TESCREAL as a conceptual framework marks a significant milestone in our understanding of the ideological underpinnings driving technological innovation, particularly in the realm of artificial intelligence. This acronym, coined by computer scientist Timnit Gebru and philosopher Émile P. Torres, encapsulates a constellation of interconnected philosophies that have profoundly shaped the trajectory of AI development and the broader tech landscape.
TESCREAL stands for:
Transhumanism
Extropianism
Singularitarianism
Cosmism
Rationalism
Effective Altruism
Longtermism
These ideologies, while distinct, share common threads and historical roots that can be traced back to the 20th century. They collectively represent a techno-optimistic worldview that envisions a future where humanity transcends its current limitations through technological advancement.
The origins of TESCREAL can be understood as a natural evolution of human aspirations in the face of rapid technological progress.
Transhumanism, for instance, emerged in the mid-20th century as a philosophy advocating for the use of technology to enhance human physical and cognitive capabilities.
Extropianism, a more optimistic offshoot of transhumanism, emphasizes continuous improvement and the expansion of human potential.
Singularitarianism, popularized by figures like Ray Kurzweil, posits the eventual emergence of artificial superintelligence that will radically transform human civilization. This concept has gained significant traction in Silicon Valley and has been a driving force behind many AI research initiatives.
Cosmism, with its roots in Russian philosophy, adds a cosmic dimension to these ideas, envisioning humanity's future among the stars. This aligns closely with the ambitions of tech entrepreneurs like Elon Musk, who are actively pursuing space exploration and colonization.
Rationalism, as incorporated in TESCREAL, emphasizes the importance of reason and evidence-based decision-making. This philosophical approach has been particularly influential in shaping the methodologies employed in AI research and development.
Effective Altruism and Longtermism, the more recent additions to this ideological bundle, bring an ethical dimension to technological pursuits. These philosophies encourage considering the long-term consequences of our actions and maximizing positive impact on a global and even cosmic scale.
The significance of TESCREAL lies in its ability to provide a comprehensive framework for understanding the motivations and goals driving some of the most influential figures and companies in the tech industry.
Consider the following example.
A major tech company announces its ambitious goal to develop artificial general intelligence (AGI) within the next decade, framing it as a breakthrough that will "solve humanity's greatest challenges." The company's leadership, steeped in TESCREAL ideologies, envisions this AGI as a panacea for global issues ranging from climate change to economic inequality.From Dr. Gebru's perspective, this scenario raises several critical concerns:
Ethical Implications: The pursuit of AGI, driven by TESCREAL ideologies, often overlooks immediate ethical concerns in favor of speculative future benefits. This approach may neglect pressing issues of bias, fairness, and accountability in current AI systems.
Power Centralization: The development of AGI by a single company or a small group of tech elites could lead to an unprecedented concentration of power, potentially exacerbating existing social and economic inequalities.
Marginalization of Diverse Perspectives: The TESCREAL framework, rooted in a particular cultural and philosophical tradition, may not adequately represent or consider the needs and values of marginalized communities globally.
Lack of Accountability: By framing AGI development as an unquestionable good for humanity, companies may evade responsibility for the potential negative consequences of their technologies.
Neglect of Present-Day Issues: The focus on long-term, speculative outcomes may divert resources and attention from addressing immediate societal challenges that AI could help solve.
Eugenics-Adjacent Thinking: There are concerning parallels between some TESCREAL ideologies and historical eugenics movements, particularly in their techno-optimistic approach to human enhancement and societal progress.
Inadequate Safety Measures: The undefined nature of AGI makes it impossible to develop comprehensive safety protocols, potentially putting society at risk.
In this view, the TESCREAL bundle of ideologies represents a problematic framework for guiding AI development. Instead, Dr. Gebru advocates for a more grounded, ethical, and inclusive approach to AI research and development. This approach prioritizes addressing current societal issues, ensuring diverse representation in AI development, and implementing robust accountability measures for AI systems and their creators.
The Legal, Economic and Policy Risks around TESCREALism
Based on the information provided, I'll explore the anticompetitive risks, challenges in skill estimation due to AI, and potential sensitization measures that can be implemented before formal regulation. I'll include examples to illustrate these points.
Anticompetitive Risks
The rapid development of AI technologies, driven by TESCREAL ideologies, can lead to several anticompetitive risks.
Market Concentration
Companies with significant resources and access to vast amounts of data may gain an unfair advantage in AI development, potentially leading to monopolistic practices.
Example: A large tech company develops an advanced AI system for healthcare diagnostics, leveraging its extensive user data. This could make it difficult for smaller companies or startups to compete, even if they have innovative ideas.
b) Algorithmic Collusion: AI systems might inadvertently facilitate price-fixing or other anticompetitive behaviors without explicit agreements between companies.
Example: The RealPage case, where multiple landlords are accused of using the same price-setting algorithm to artificially inflate rental prices, demonstrates how AI can potentially lead to collusive behavior without direct communication between competitors[2].
Risks Around Skill "Census" and Estimation
AI's impact on the job market and skill requirements poses challenges for accurate workforce planning:
Rapid Skill Obsolescence
AI may accelerate the pace at which certain skills become outdated, making it difficult for workers and organizations to keep up.
Example: As AI takes over routine coding tasks, software developers may need to quickly shift their focus to more complex problem-solving and AI integration skills.
Skill Gap Identification
While AI can help identify skill gaps, there's a risk of over-reliance on AI-driven assessments without considering human factors.
Example: An AI system might identify a need for data analysis skills in a company but fail to recognize the importance of domain expertise or soft skills that are crucial for interpreting and communicating the results effectively.
Sensitization Measures Before Regulation
To address these challenges before formal regulation is implemented, several sensitization measures can be considered:
Promote Explainable AI (XAI)
Encourage the development of AI systems that can provide clear explanations for their decisions. This can help identify potential biases or anticompetitive behaviors.
Example: Implement a requirement for AI-driven hiring systems to provide explanations for candidate rankings or rejections, allowing for human oversight and intervention.
Foster Multi-stakeholder Dialogue
Create forums for discussion between industry leaders, policymakers, academics, and civil society to address potential risks and develop best practices.
Example: Organize regular roundtable discussions or conferences where AI developers, ethicists, and labor representatives can discuss the impact of AI on workforce dynamics and potential mitigation strategies.
Encourage Voluntary Ethical Guidelines
Promote the adoption of voluntary ethical guidelines for AI development and deployment within industries.
Example: Develop an industry-wide code of conduct for AI use in financial services, addressing issues such as algorithmic trading and credit scoring.
Invest in AI Literacy Programs
Develop educational initiatives to improve public understanding of AI capabilities, limitations, and potential impacts.
Example: Create online courses or workshops for employees and the general public to learn about AI basics, its applications, and ethical considerations.
Support Adaptive Learning and Reskilling Initiatives
Encourage companies to invest in continuous learning programs that help employees adapt to AI-driven changes in the workplace.
Example: Implement AI-powered adaptive learning platforms that personalize training content based on individual skill gaps and learning speeds[7].
Promote Transparency in AI Development
Encourage companies to be more transparent about their AI development processes and potential impacts on the workforce and market dynamics.
Example: Implement voluntary reporting mechanisms where companies disclose their AI use cases, data sources, and potential societal impacts.
How does our AIACT.IN proposal address AI hype and the effect of TESCREALism
Here are some key features related to sensitisation measures, anticompetitive risks, and skill estimation:
Enhanced Classification Methods: The draft introduces more nuanced and precise evaluation methods for AI systems, considering conceptual, technical, commercial, and risk-centric approaches. This allows for better risk management and tailored regulatory responses.
National Registry for AI Use Cases: A comprehensive framework for tracking both untested and stable AI applications across India, promoting transparency and accountability.
AI-Generated Content Regulation: Balances innovation with protection of individual rights and societal interests, including content provenance requirements like watermarking.
Advanced AI Insurance Policies: Manages risks associated with high-risk AI systems to ensure adequate protection for stakeholders.
AI Pre-classification: Enables early assessment of potential risks and benefits of AI systems.
Guidance on AI-related Contracts: Provides principles for responsible AI practices within organizations, addressing potential anticompetitive concerns.
National AI Ethics Code: Establishes a flexible yet robust ethical foundation for AI development and deployment.
Interoperability and Open Standards: Encourages adoption of open standards and interoperability in AI systems, potentially lowering entry barriers and promoting competition.
Algorithmic Transparency: Requires maintaining records of algorithms and data used to train AI systems, aiding in detecting bias and anti-competitive practices.
Thanks for reading this insight.
Since May 2024, we have launched some specialised practice-based technology law and artificial intelligence & law training programmes at indicpacific.com/train.
We offer special discounts for technology teams who may be interested in enrolling for the training programmes on bulk. Feel free to choose your training programme at indicpacific.com/train and contact us at vligta@indicpacific.com.
Comments