Indo-Pacific Research Principles on Use of Large Reasoning Models & 2 Years of IndoPacific.App
- Communications Team
- 3 days ago
- 5 min read
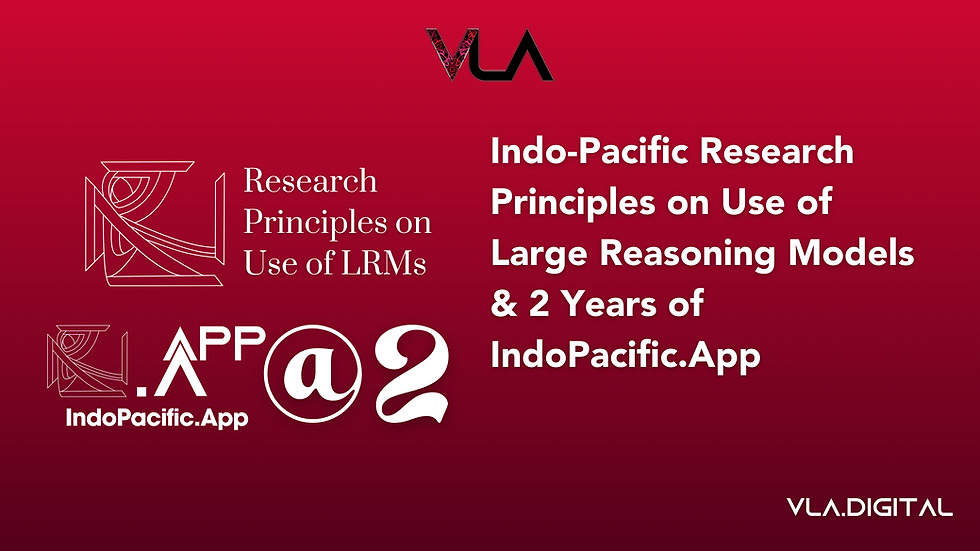
The firm is delighted to first announce the launch of Indo Pacific Research Principles on Large Reasoning Models, and also announces two successful years of IndoPacific App, our legal and policy literature archive since 2023. The first section covers the firm's reasoning to introduce these principles on large reasoning models.
The Research Principles: Their Purpose
Now, Large Reasoning Models as developed by AI companies across the globe, whose examples include Deeper Search & Deep Research by XAI (Grok), Deep Research by Perplexity, Gemini's Deep Research and even OpenAI's own Deep Reasoning tool, are supposed to mimic reasoning abilities of human beings. The development of LRMs emerged from the recognition that standard LLMs often struggle with complex reasoning tasks despite their impressive language generation capabilities. Researchers observed, or say, supposed, that prompting LLMs to "think step by step" or to break down problems into smaller components often improved performance on mathematical, logical, and algorithmic challenges.
Models like DeepSeek R1, Claude, and GPT-4 are frequently cited examples that incorporate reasoning-focused architectures or training methodologies. These models are trained to produce intermediate steps that suppose to 'resemble' human reasoning processes before arriving at final answers.
These models, which include systems like Claude 3.7, DeepSeek R1, GPT-4, and others, claim to exhibit reasoning capabilities that mimic human thought processes, often displaying their work through "reasoning traces" or step-by-step explanations of their thought processes. However, recent research has begun to question these claims and identify significant limitations in how these models actually reason.
While LRMs have shown some performance on certain benchmarks, researchers have found substantial evidence suggesting that what appears to be reasoning may actually be sophisticated pattern matching rather than genuine logical processing.
The Anthropomorphisation Trap
A critical issue in evaluating LRMs is what researchers call the "anthropomorphisation trap" - the tendency to interpret model outputs as reflecting human-like reasoning processes simply because they superficially resemble human thought patterns. The inclusion of phrases like "hmm..," "aha..," "let me think step by step..," may create the impression of deliberative thinking, but these are more likely stylistic imitations of human reasoning patterns present in training data rather than evidence of actual reasoning.
This trap is particularly concerning because it can lead researchers and users to overestimate the models' capabilities. When LRMs produce human-like reasoning traces that appear thoughtful and deliberate, we may incorrectly attribute sophisticated reasoning abilities to them that don't actually exist.
Here is a table that gives you an overview of the limitations associated with large reasoning models.
Reasoning Limitation in Large Reasoning Models | Description |
Lack of True Understanding | LRMs operate by predicting the next token based on patterns they've learned during training, but they fundamentally lack a deep understanding of the environment and concepts they discuss. This limitation becomes apparent in complex reasoning tasks that demand true comprehension rather than pattern recognition. |
Contextual and Planning Limitations | Although modern language models excel at grasping short contexts, they often struggle to maintain coherence over extended conversations or larger text segments. This can result in reasoning errors when the model must connect information from various parts of a dialogue or text. Additionally, LRMs frequently demonstrate an inability to perform effective planning for multi-step problems. |
Deductive vs. Inductive Reasoning | Research indicates that LRMs particularly struggle with deductive reasoning, which requires deriving specific conclusions from general principles with a high degree of certainty and logical consistency. Their probabilistic nature makes achieving true deductive closure difficult, creating significant limitations for applications requiring absolute certainty. |
Even in the paper co-authored by Prof. Subbarao Kambhampati, entitled, "A Systematic Evaluation of the Planning and Scheduling Abilities of the Reasoning Model o1" directly addresses critical themes from our earlier discussion about Large Reasoning Models (LRMs). Here are some quotes from this paper:
While o1-mini achieves 68% accuracy on IPC domains compared to GPT-4's 42%, its traces show non-monotonic plan construction patterns inconsistent with human problem-solving [...] At equivalent price points, iterative LLM refinement matches o1's performance, questioning the need for specialized LRM architectures. [...] Vendor claims about LRM capabilities appear disconnected from measurable reasoning improvements.
The Indo-Pacific Research Principles on Use of Large Reasoning Models
Based on the evidence we have collected, and the insights received, we have proposed the following research principles on use of large reasoning models by Indic Pacific Legal Research.
Principle 1: Emphasise Formal Verification
Train LRMs to produce verifiable reasoning traces, like A* dynamics or SoS, for rigorous evaluation.
Principle 2: Be Cautious with Intermediate Traces
Recognise traces may be misleading; do not rely solely on them for trust or understanding.
Principle 3: Avoid Anthropomorphisation
Focus on functional reasoning, not human-like traces, to prevent false confidence.
Principle 4: Evaluate Both Process and Outcome
Assess both final answer accuracy and reasoning process validity in benchmarks.
Principle 5: Transparency in Training Data
Be clear about training data, especially human-like traces, to understand model behaviour.
Principle 6: Modular Design
Use modular components for flexibility in reasoning structures and strategies.
Principle 7: Diverse Reasoning Structures
Experiment with chains, trees, graphs for task suitability, balancing cost and effectiveness.
Principle 8: Operator-Based Reasoning
Implement operators (generate, refine, prune) to manipulate and refine reasoning processes.
Principle 9: Balanced Training
Use SFT and RL in two-phase training for foundation and refinement.
Principle 10: Process-Based Evaluation
Evaluate the entire reasoning process for correctness and feedback, not just outcomes.
Principle 11: Integration with Symbolic AI
Combine LRMs with constraint solvers or planning algorithms for enhanced reasoning.
Principle 12: Interactive Reasoning
Design LRMs for environmental interaction, using feedback to refine reasoning in sequential tasks.
Please note that all principles are purely consultative and constitute no binding value on the members of Indic Pacific Legal Research. We also permit the use of these principles, provided that we are well-cited and referenced, for strict non-commercial use.
IndoPacific.App Celebrates its Glorious 2 Years

The IndoPacific App, launched under Abhivardhan's leadership in 2023 was a systemic reform undergone at Indic Pacific Legal Research to document our research publications, and contributions better. In our partnership with the Indian Society of Artificial Intelligence and Law, ISAIL.IN's publications, and documentations are also registered at the IndoPacific App, under the AiStandard.io Alliance, and even otherwise as well.
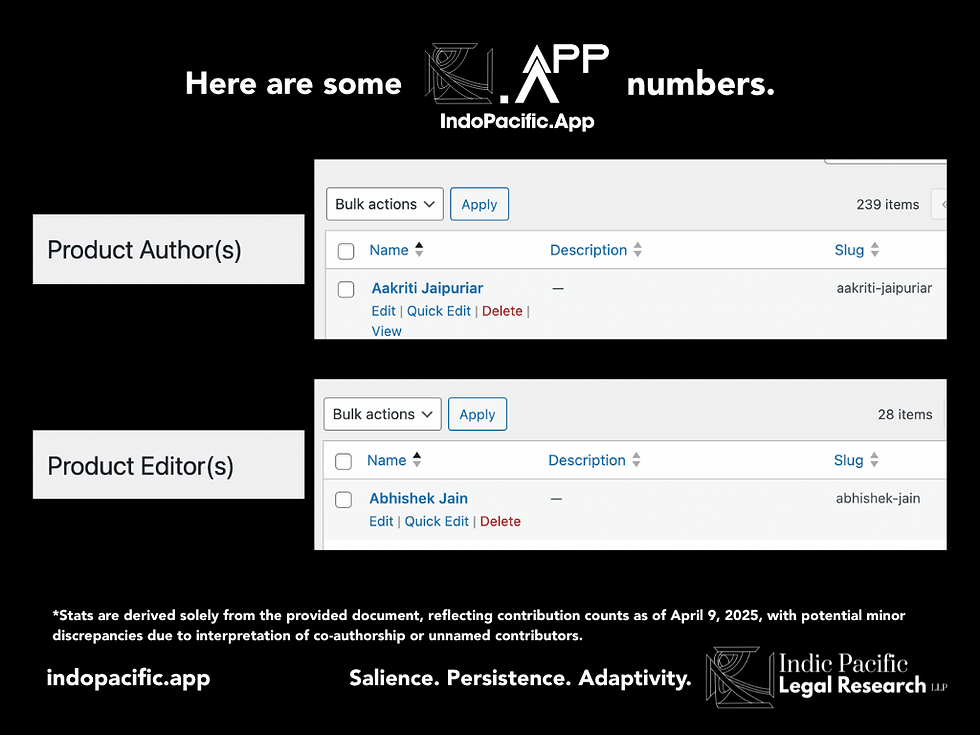
As this archive of legal (mostly) and policy literature completes its 2 years of existence under Indic Pacific's VLiGTA research & innovation division, we are glad to put some statistics clear for everyone's purview, which is updated as of April 12, 2025, and verified by manual means, after our use of Generative AI tools. This means that the stats is double-checked:
We host publications and documentations of exactly 238 original authors (1 error removed).
Our founder, Mr Abhivardhan's own publications constitute around 10% (approx.)
The number of publications on IndoPacific App stand at 85, however, the number of chapters or contributory sections or articles, if we add numbers within research collections, then the number of research contributions stand at 304 unique contributions, which is a historic figure.
Now, if we attribute these 304 unique contributions to each author (which are in the form of chapters to a collection of research or handbook, or a report, or a brief, for instance) - then the number of individual author credits will cross 300 as per our approximate estimates.
This means something simple, honest and obvious.
The IndoPacific.App, started by our Founder, Abhivardhan, is the biggest technology law archive of mostly Indian authors, with around 238 original authors documented in this archive, and 304 unique contributions (published) featured.
There is no law firm, consultancy, think tank or institution with such a huge technology law archive, with independent support, and we are proud to have achieved this feat in the 5 years span of existence of both Indic Pacific Legal Research, and the Indian Society of Artificial Intelligence and Law.
Thank you for becoming a part of this research community either through Indic Pacific Legal Research, or the Indian Society of Artificial Intelligence and Law. It is our honour and duty to safeguard this archive for all, which is 99% (except the handbooks) free.
So, don't wait, go and download some amazing works from the IndoPacific.app, today.
Comments