Beyond the AI Garage: India's New Foundational Path for AI Innovation + Governance in 2025
- Abhivardhan
- Jan 22
- 21 min read
Updated: Jan 24
This is quite a long read.
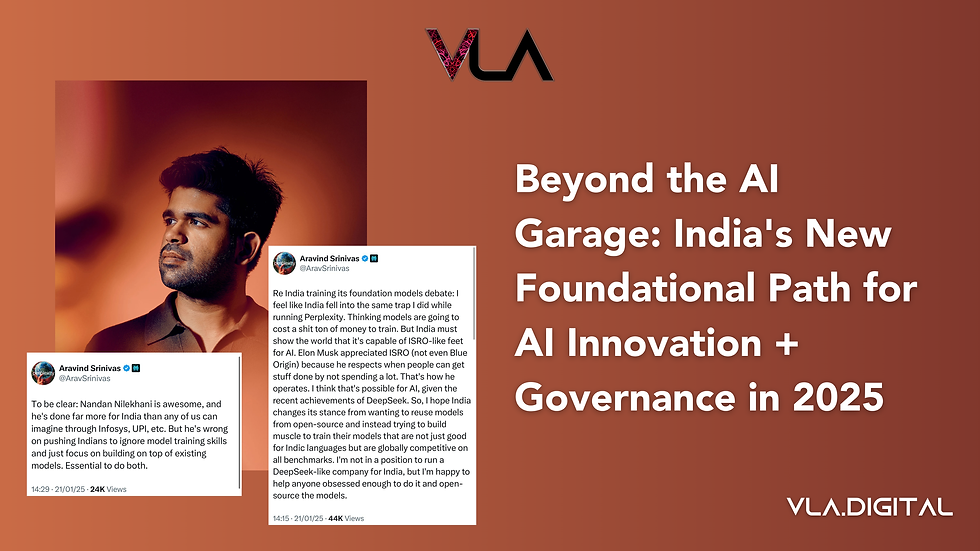
India's artificial intelligence landscape stands at a pivotal moment, where critical decisions about model training capabilities and research directions will shape its technological future. The discourse was recently energised by Aravind Srinivas, CEO of Perplexity AI, who highlighted two crucial perspectives that challenge India's current AI trajectory.
The Wake-up Call
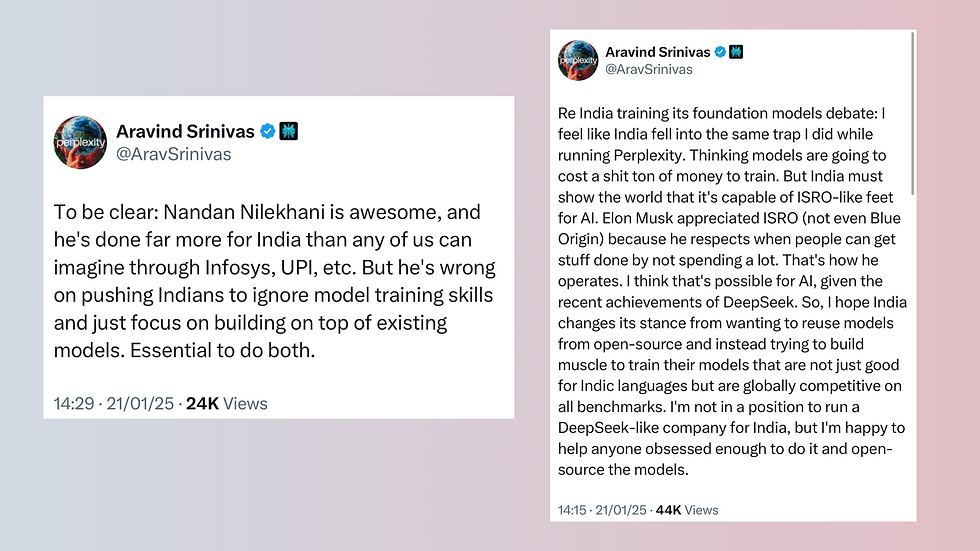
Srinivas emphasises that India's AI community faces a critical choice: either develop model training capabilities or risk becoming perpetually dependent on others' models. His observation that "Indians cannot afford to ignore model training" stems from a deeper understanding of the AI value chain. The ability to train models represents not just technical capability, but technological sovereignty.
A significant revelation comes from DeepSeek's recent achievement. Their success in training competitive models with just 2,048 GPUs challenges the widespread belief that model development requires astronomical resources. This demonstrates that with strategic resource allocation and expertise, Indian organisations can realistically pursue model training initiatives.
India's AI ecosystem currently focuses heavily on application development and use cases. While this approach has yielded short-term benefits, it potentially undermines long-term technological independence. The emphasis on building applications atop existing models, while important, shouldn't overshadow the need for fundamental research and development capabilities.
In short, Srinivas attempts to highlight 3 key issues, through his posts, on the larger tech development and application layer debate in India:
Limited hardware infrastructure for AI model training
Concentration of model training expertise in select global companies
Over-reliance on foreign AI models and frameworks
This insight fixates itself on legal and policy perspectives around building necessary capabilities around innovating in core AI models, and also focusing on building use case capitals in India, including in Bengaluru and other places. In addition, this long insight covers recommendations to the Ministry of Electronics and Information Technology, Government of India on the Report on AI Governance Guidelines Development, in the concluding sections.
The Policy Imperative: Balancing Use Cases and Foundational AI Development
What is pointed out by Aravind Srinivas about AI development avenues in India's scenario is also backed by policy & industry realities. The recent repeal of the former Biden Administration (US Government)'s Executive Order on Artificial Intelligence by the Trump Administration hours ago demonstrated that the US Government's focus has pivoted on hard resource considerations around AI development, such as data centres, semiconductors, and talent. India has no choice but to keep both ideas - building use case capitals in India, and focus on foundational AI research alternatives, at the same time.
Moving Beyond Use-Case Capitalism
India's current AI strategy has been heavily skewed toward application development—leveraging existing foundational models like those from OpenAI or Google to build domain-specific use cases. While this approach has yielded quick wins in sectors like healthcare, agriculture, and governance, it risks creating along-term dependency on foreign technologies. This"use-case capitalism" prioritises short-term gains over the strategic imperative of building indigenous capabilities.
Technological Dependence: Relying on pre-trained foundational models developed abroad limits India's ability to innovate independently and negotiate favourable terms in the global AI ecosystem.
Economic Vulnerability: By focusing on applications rather than foundational research, India risks being relegated to a secondary role in the AI value chain, capturing only a fraction of the economic value generated by AI technologies.
Missed Opportunities for Sovereignty: Foundational models are critical for ensuring control over data, algorithms, and intellectual property. Without them, India remains vulnerable to external control over critical AI infrastructure.
Building Indigenous Model Training Capabilities
The ability to train foundational models domestically is essential for achieving technological independence. Foundational models—large-scale pre-trained systems—are the backbone of generative AI applications. Building such capabilities in India requires addressing key gaps in infrastructure, talent, and data availability.
Key Challenges
Infrastructure Deficit:
Training large-scale models requires significant computational resources (e.g., GPUs or TPUs). India's current infrastructure lags behind global leaders like the US and China.
Initiatives like CDAC’s AIRAWAT supercomputer are steps in the right direction but need scaling up.
Talent Shortage:
While India has a large tech workforce (420,000 professionals), expertise in training large language models (LLMs) remains concentrated in a few institutions like IITs and IISc.
Collaboration with global experts and targeted upskilling programs are necessary to bridge this gap.
Data Limitations:
High-quality datasets for Indian languages are scarce, limiting the ability to train effective multilingual models.
Efforts like Bhashini have made progress but need expansion to include diverse domains such as agriculture, healthcare, and governance.
From Lack of Policy Clarity to AI Safety Diplomacy
The Indian government has positioned itself as a participant in the global conversation on artificial intelligence (AI) regulation, emphasising its leadership on equity issues relevant to the Global South while proposing governance frameworks for AI. However, these initiatives often appear inconsistent and lack coherence.
For instance, in April 2023, former Minister of State of Electronics & Information Technology, Rajeev Chandrasekhar had asserted that India would not regulate AI, aiming to foster a pro-innovation environment. Yet, by June of the same year, he shifted his stance, advocating for regulations to mitigate potential harms to users. India’s approach to AI regulation is thereby fragmented and overreactive, with overlapping initiatives and strategies across various government entities.
Now, we have to understand 2 important issues here.
The AI-related approaches, and documents adopted by statutory bodies, regulators, constitutional authorities under Article 324 of the Indian Constitution and even non-tech ministries have some specificity and focus, as compared to MeiTY. For example, the Election Commission of India came up with an advisory on content provenance of any synthetic content/ deepfakes being used during election campaigning.
MeiTY, on the other hand, had been rushing on AI governance initiatives, thanks to the Advisory they had published on March 1, 2024, replaced by a subsequent advisory in the 2nd week of March of the same year. By October 2024, however, the Government of India had pivoted its approach towards AI regulation in 2 facets: (1) the Principle Scientific Advisor's Office had taken over major facets of AI regulation policy conundrums; and (2) MeiTY narrows down its goals of AI regulation to AI Safety by considering options to develop an Artificial Intelligence Safety Institute.
Unlike many law firms, chambers and think tanks who might have been deceptive in their discourse on India's AI regulation & data protection landscape, the reality is simple that the Government of India keeps publishing key AI industry, and policy / governance guidelines without showing any clear intent to regulate AI per se.
The focus towards over-regulation / regulatory capture in the field of artificial intelligence doesn't exist. In fact, the Government has taken a patient approach by stating that some recognition of key liability issues around certain mainstream AI applications (like deepfakes) can be addressed by tweaking existing criminal law instruments. Here's what was quoted from S Krishnan's statement at the Global AI Conclave of end-November 2024.
Deepfakes is not a regulatory issue, it is a technology issue. However we may need few tweaks in regulations, as opposed to a complete overhaul.
In fact, this statement by MeiTY Secretary S Krishnan, is truly appreciative:
Intellectual Property (IP) Challenges: Wrappers and Foundational Models
The reliance on "wrappers"—deliverables or applications built on top of existing foundational AI models—raises significant intellectual property (IP) concerns. These challenges are particularly pronounced in the Indian context, where businesses and end-users face risks associated with copyright, trade secrets, and patentability.
Copyright Issues with AI-Generated Content
AI-generated content, often used in wrappers, presents a fundamental challenge to traditional copyright frameworks.
Lack of Ownership Clarity: Determining ownership of AI-generated content is contentious. For example, does the copyright belong to the developer of the foundational model, the user providing input prompts, or the organisation deploying the wrapper? This ambiguity undermines enforceability.
Attribution Gaps: Foundational models often use vast datasets without proper attribution during training. This creates potential liabilities for businesses using wrappers built on these models if outputs closely resemble copyrighted material.
These uncertainties make it difficult for Indian businesses to claim exclusive rights over wrapper-based deliverables, exposing them to potential legal disputes and economic risks.
Trade Secrets and Proprietary Model Risks
Trade secrets are critical for protecting proprietary algorithms, datasets, and other confidential information embedded within foundational models. However, wrappers built on these models face unique vulnerabilities:
Reverse Engineering: Competitors can potentially reverse-engineer wrappers to uncover proprietary algorithms or techniques from the foundational models they rely on. This compromises the confidentiality essential for trade secret protection.
Data Security Threats: Foundational models often retain input data for further training or optimization. Wrappers that interface with such models risk exposing sensitive business data to unauthorised access or misuse.
Algorithmic Biases: Biases embedded in foundational models can inadvertently compromise trade secret protections by revealing patterns or vulnerabilities during audits or legal disputes.
Insider Threats: Employees with access to wrappers and underlying foundational models might misuse confidential information, especially in industries with high turnover rates.
These risks are exacerbated by India's lack of a dedicated trade secret law, relying instead on common law principles and contractual agreements like non-disclosure agreements (NDAs).
Patentability Challenges
The patent system in India poses significant hurdles for innovations involving foundational models and their wrappers due to restrictive interpretations under Section 3(k) of the Patents Act, 1970. Key challenges include:
Subject Matter Exclusions: Algorithms and computational methods integral to foundational models are excluded from patent eligibility unless they demonstrate a "technical effect." This limits protections for innovations derived from these models.
Inventorship Dilemmas: Indian patent law requires inventors to be natural persons. This creates a legal vacuum when foundational models autonomously generate novel solutions integrated into wrappers.
Global Disparities: While jurisdictions like the U.S. and EU have begun adapting their patent frameworks for AI-related inventions, India's outdated approach discourages investment in foundational model R&D.
Economic Risks: Without clear patent protections, Indian businesses may struggle to attract funding for wrapper-based innovations that rely on foundational model advancements.
These challenges highlight the systemic barriers preventing Indian innovators from fully leveraging foundational models while protecting their intellectual property.
Negotiation Leverage Over Foundational Models
Indian businesses relying on foreign-owned foundational models face additional risks tied to access rights and licensing terms:
Restrictive Licensing Agreements: Multinational corporations (MNCs) controlling foundational models often impose restrictive terms that limit customization or repurposing by Indian businesses.
Data Ownership Conflicts: Foundational models trained on Indian datasets may not grant reciprocal rights over outputs generated using those datasets, creating an asymmetry in value capture.
Supply Chain Dependencies: Dependence on global digital value chains exposes Indian businesses to geopolitical risks, price hikes, or service disruptions that could impact access to critical AI infrastructure.
These legal-policy issues are critical and cannot be ignored by the Government of India, nor by major Indian companies, emerging AI companies, as well as research labs & other market cum technical stakeholders.
The Case for a CERN-Like Body for AI Research: Moving Beyond the "AI Garage"
India's current positioning as an "AI Garage" for developing and emerging economies, as outlined in its AI strategy of 2018, emphasizes leveraging AI to solve practical, localized problems. While this approach has merit in addressing immediate societal challenges, it risks limiting India's role to that of an application developer rather than a leader in foundational AI research. To truly establish itself as a global AI powerhouse, India must advocate for and participate in the creation of a CERN-like body for artificial intelligence research.
The Limitations of the "AI Garage" Approach
The "AI Garage" concept, promoted by NITI Aayog, envisions India as a hub for scalable and inclusive AI solutions tailored to the needs of emerging economies. While this aligns with India's socio-economic priorities, it inherently focuses on downstream applications rather than upstream foundational research. This approach creates several limitations:
Dependence on Foreign Models: By focusing on adapting existing foundational models (developed by global tech giants like OpenAI or Google), India remains dependent on external technologies and infrastructure.
Missed Opportunities for Leadership: The lack of investment in foundational R&D prevents India from contributing to groundbreaking advancements in AI, relegating it to a secondary role in the global AI value chain.
Limited Global Influence: Without leadership in foundational research, India's ability to shape global AI norms, standards, and governance frameworks is diminished.
The Vision for a CERN-Like Body
A CERN-like body for AI research offers an alternative vision—one that emphasizes international collaboration and foundational R&D. Gary Marcus, a prominent AI researcher and critic of current industry practices, has long advocated for such an institution since 2017. He argues that many of AI's most pressing challenges—such as safety, ethics, and generalization—are too complex for individual labs or profit-driven corporations to address effectively. A collaborative body modeled after CERN (the European Organization for Nuclear Research) could tackle these challenges by pooling resources, expertise, and data across nations.
Key features of such a body include:
Interdisciplinary Collaboration: Bringing together experts from diverse fields such as computer science, neuroscience, ethics, and sociology to address multifaceted AI challenges.
Open Research: Ensuring that research outputs, datasets, and foundational architectures are publicly accessible to promote transparency and equitable benefits.
Focus on Public Good: Prioritising projects that address global challenges—such as climate change, healthcare disparities, and education gaps—rather than narrow commercial interests.
Why India Needs to Lead or Participate
India is uniquely positioned to champion the establishment of a CERN-like body for AI due to its growing digital economy, vast talent pool, and leadership in multilateral initiatives like the Global Partnership on Artificial Intelligence (GPAI). However, if the United States remains reluctant to pursue such an initiative on a multilateral basis, India must explore partnerships with other nations like the UAE or Singapore.
Strategic Benefits for India:
Anchor for Foundational Research: A CERN-like institution would provide India with access to cutting-edge research infrastructure and expertise.
Trust-Based Partnerships: Collaborative research fosters trust among participating nations, creating opportunities for equitable technology sharing.
Global Influence: By playing a central role in such an initiative, India can shape global AI governance frameworks and standards.
Why UAE or Singapore Could Be Viable Partners:
The UAE has already demonstrated its commitment to becoming an AI leader through initiatives like its National Artificial Intelligence Strategy 2031. Collaborating with India would align with its policy goals while providing access to India's talent pool.
Singapore's focus on innovation-driven growth makes it another strong candidate for partnership. Its robust digital infrastructure complements India's strengths in data and software development.
The Need for Large-Scale Collaboration
As Gary Marcus has pointed out, current approaches to AI research are fragmented and often driven by secrecy and competition among private corporations. This model is ill-suited for addressing fundamental questions about AI safety, ethics, and generalization. A CERN-like body would enable large-scale collaboration that no single nation or corporation could achieve alone. For example:
AI Safety: Developing frameworks to ensure that advanced AI systems operate reliably and ethically across diverse contexts.
Generalization: Moving beyond narrow task-specific models toward systems capable of reasoning across domains.
Equitable Access: Ensuring that advancements in AI benefit all nations rather than being concentrated in a few tech hubs.
India's current "AI Garage" approach is insufficient if the country aims to transition from being a consumer of foundational models to a creator of them. Establishing or participating in a CERN-like body for AI research represents a transformative opportunity—not just for India but also for the broader Global South.
The Case Against an All-Comprehensive AI Regulation in India
As the author of this insight has also developed India's first privately proposed AI regulation, aiact.in, the experience and feedback from stakeholders has been that a sweeping, all-encompassing AI Act is not the right course of action for India at this moment. While the rapid advancements in AI demand regulatory attention, rushing into a comprehensive framework could lead to unintended consequences that stifle innovation and create more confusion than clarity.
Why an AI Act is Premature
India’s AI ecosystem is still in its formative stages, with significant gaps in foundational research, infrastructure, and policy coherence. Introducing a broad AI Act now risks overregulating an industry that requires flexibility and room for growth. Moreover:
Second-Order Effects: Feedback from my work on AI regulation highlights how poorly designed laws can have ripple effects on innovation, investment, and adoption. For example, overly stringent rules could discourage startups and SMEs from experimenting with AI solutions.
Sectoral Complexity: The diverse applications of AI—ranging from healthcare to finance—demand sector-specific approaches rather than a one-size-fits-all regulation.
Recommendations on the Report on AI Governance Guidelines Development by IndiaAI of January 2025
Part 1: Feedback on the AI Governance Principles as proposed and stated to align with OECD, NITI and NASSCOM's efforts.
Principle | Feedback |
Transparency: AI systems should be accompanied with meaningful information on their development, processes, capabilities & limitations, and should be interpretable and explainable, as appropriate. Users should know when they are dealing with AI. | The focus on interpretability & explainability with a sense of appropriate considerations, is appreciated. |
Accountability: Developers and deployers should take responsibility for the functioning and outcomes of AI systems and for the respect of user rights, the rule of law, & the above principles. Mechanisms should be in place to clarify accountability. | The principle remains clear with a pivotal focus on rule of law & the respect of user rights, and is therefore appreciated. |
Safety, reliability & robustness: AI systems should be developed, deployed & used in a safe, reliable, and robust way so that they are resilient to risks, errors, or inconsistencies, the scope for misuse and inappropriate use is reduced, and unintended or unexpected adverse outcomes are identified and mitigated. AI systems should be regularly monitored to ensure that they operate in accordance with their specifications and perform their intended functions. | The reference to acknowledge the link between any AI system's intended functions (or intended purpose) and specifications, with safety, reliability and robustness considerations is appreciated. |
Privacy & security: AI systems should be developed, deployed & used in compliance with applicable data protection laws and in ways that respect users’ privacy. Mechanisms should be in place to ensure data quality, data integrity, and ‘security-by-design’. | The indirect reference via the term 'security-by-design' is to the security safeguards under the National Data Management Office framework under the IndiaAI Expert Group Report of October 2023, and the Digital Personal Data Protection Act, 2023, in spirit. This is also appreciated. |
Fairness & non-discrimination: AI systems should be developed, deployed, & used in ways that are fair and inclusive to and for all and that do not discriminate or perpetuate biases or prejudices against, or preferences in favour of, individuals, communities, or groups. | The principle's wording seems fine, but it could have also emphasised upon technical biases, and not just biases causing socio-economic or socio-technical disenfranchisement or partiality. Otherwise, the wording is pretty decent & normal. |
Human-centred values & ‘do no harm’: AI systems should be subject to human oversight, judgment, and intervention, as appropriate, to prevent undue reliance on AI systems, and address complex ethical dilemmas that such systems may encounter. Mechanisms should be in place to respect the rule of law and mitigate adverse outcomes on society. | The reference to the phrase 'do no harm' is the cornerstone of this principle, in context of what appropriate human oversight, judgment and intervention may be feasible. Since this is a human-centric AI principle, the reference to 'adverse outcomes on society' was expected, and is appreciated. |
Inclusive & sustainable innovation: The development and deployment of AI systems should look to distribute the benefits of innovation equitably. AI systems should be used to pursue beneficial outcomes for all and to deliver on sustainable development goals. | The distributive aspect of innovation benefits that the development and deployment of AI systems may be agreed as a generic international law principle to promote AI Safety Research, and evidence-based policy making & diplomacy. |
Digital by design governance: The governance of AI systems should leverage digital technologies to rethink and re-engineer systems and processes for governance, regulation, and compliance to adopt appropriate technological and techno-legal measures, as may be necessary, to effectively operationalise these principles and to enable compliance with applicable law. | This principle suggests that the infusion of AI governance by default should be driven by digital technologies by virtue of leverage. It is therefore recommended that the reference to "technological and techno-legal measures" is not viewed as solution-obsessed AI governance measure. Compliance technologies cannot replace technology law adjudication and human autonomy, which is why despite using the word 'appropriate', the reference to the 'measures' seems solution-obsessed, because obsessing with solution-centricity does not address issues of technology maintenance & efficiency by default. Maybe the intent to promote 'leverage' and 'rethink' should be considered as a consultative aspect of the principle, and not a fundamental one. |
Part B: Feedback on Considerations to operationalise the principles
Examining AI systems using a lifecycle approach
While governance efforts should keep the lifecycle approach as an initial consideration, the ecosystem view of AI actors should take precedence over all considerations to operationalise the principles.
The best reference to the lifecycle approach can be technical, and management-centric, about best practices, and not about policy. As stated in the document, the lifecycle approach should merely be considered 'useful', and nothing else.
The justification of 'Diffusion' as a stage is very unclear, since "examining the implications of multiple AI systems being widely deployed and used across multiple sectors and domains" by default should be specific to the intended purpose of AI systems and technologies. Some tools or applications might not have a multi-sectoral effect.
Therefore, 'Diffusion' by virtue of its meaning should be considered as an add-on stage. In fact the reference to Diffusion would only suit General Purpose AI systems, if we take the OECD and European Union definitions of General Purpose AI systems into consideration. This should either be an add-on phase, or should be taken into direct correlation with how intended purpose of AI systems is banked upon. Otherwise, the reference to this phase may justify regulatory capture.
Taking an ecosystem-view of AI actors
The listing of actors as a matter of exemplification in the context of foundation model seems appropriate. However, in the phrase "distribution of responsibilities and liabilities", the term distribution should be replaced with apportionment, and the phrase "responsibilities and liabilities" should be replaced with accountability and responsibility only. Here is an expanded legal reasoning, put forth:
Precision in Terminology: The term distribution implies an equal or arbitrary allocation of roles and duties, which may not accurately reflect the nuanced and context-specific nature of governance within an AI ecosystem. Apportionment, on the other hand, suggests a deliberate and proportional assignment of accountability and responsibility based on the roles, actions, and influence of each actor within the ecosystem. This distinction is critical in ensuring that governance frameworks are equitable and just.
Legal Clarity on Liability: The inclusion of liabilities in the phrase "responsibilities and liabilities" introduces ambiguity, as liability is not something that can be arbitrarily allocated or negotiated among actors. Liability regimes are determined by statutory provisions and case law precedents, which rely on existing legal frameworks rather than ecosystem-specific governance agreements. Therefore, retaining accountability and responsibility—terms that are more flexible and operational within governance frameworks—avoids conflating governance mechanisms with legal adjudication.
Role of Statutory Law: Liability pertains to legal consequences that arise from breaches or harms, which are adjudicated based on statutory laws or judicial interpretations. Governance frameworks cannot override or preempt these legal determinations but can only clarify roles to ensure compliance with existing laws. By focusing on accountability and responsibility, the framework aligns itself with operational clarity without encroaching upon the domain of statutory liability.
Ecosystem-Specific Context: Foundation models involve multiple actors—developers, deployers, users, regulators—each with distinct roles. Using terms like apportionment emphasizes a tailored approach where accountability is assigned proportionally based on each actor's influence over outcomes, rather than a blanket distribution that risks oversimplification.
Avoiding Overreach: Governance frameworks should aim to clarify operational responsibilities without overstepping into areas governed by statutory liability regimes. This ensures that such frameworks remain practical tools for managing accountability while respecting the boundaries set by law.
Leveraging technology for governance
The conceptual approach outlined in the provided content, while ambitious and forward-looking, suffers from several critical weaknesses that undermine its practicality and coherence:
Overly Broad and Linguistically Ambiguous: The conceptual approach is excessively verbose and broad, which dilutes its clarity and focus. The lack of precision in defining key terms, such as "techno-legal approach," creates interpretative ambiguity. This undermines its utility as a concrete governance framework and risks being dismissed as overly theoretical or impractical.
Misplaced Assumption About Techno-Legal Governance: The assertion that a "complex ecosystem of AI models, systems, and actors" necessitates a techno-legal approach is unsubstantiated. The government must clarify whether this approach is a solution-obsessed strategy focused on leveraging compliance technology as a panacea for governance challenges. If so, this is deeply flawed because:
It assumes technology alone can address systemic issues without adequately considering the socio-political and institutional capacities required for effective implementation.
It risks prioritizing tools over outcomes, leading to a governance framework that is reactive rather than adaptive.
Counterproductive Supplementation of Legal Regimes: Merely supplementing legal and regulatory regimes with "appropriate technology layers" is insufficient to oversee or promote growth in a rapidly expanding AI ecosystem. This mirrors the whole-of-government approach proposed under the Digital India Act (of March 2023) but lacks the necessary emphasis on capacity-building among legal and regulatory institutions. Without consistent capacity-building efforts:
The techno-legal approach remains an abstract concept with no actionable pathway.
It risks becoming verbal gibberish that fails to translate into meaningful governance outcomes.
Practical Flaws in Risk Mitigation and Compliance Automation: While using technology to mitigate risks, scale, and automate compliance appears conceptually appealing, it is fraught with practical challenges:
Risk estimation, scale estimation, and compliance objectives are not standardised across sectors or jurisdictions in India.
Most government agencies, regulatory bodies, and judicial authorities lack the technical expertise to understand or implement automation effectively.
There is no settled legal or technical framework to support AI safety measures in a techno-legal manner. This undermines evidence-based policymaking and contradicts the ecosystem view of AI actors by failing to account for their diverse roles and responsibilities.
As a result, this aspect of the conceptual approach is far-fetched and impractical in India's current regulatory landscape.
Unrealistic Assumptions About Liability Allocation: The proposal to use technology for allocating regulatory obligations and apportioning liability across the AI value chain is premature and lacks empirical support. According to the Ada Lovelace Institute, assigning responsibilities and liabilities in AI supply chains is particularly challenging due to the novelty, complexity, and rapid evolution of AI systems. To add further:
No country has successfully implemented such a system, not even technologically advanced nations like the US, China, or UAE.
The availability of sufficient data and content to process such allocations remains uncertain.
Terms like "lightweight but gradually scalable" are misleading in the context of India's complex socio-economic fabric. Without robust evidence-based policymaking or AI safety research, this proposal risks being overly optimistic and disconnected from ground realities.
Overreach in Proposing Tech Artefacts for Liability Chains: The suggestion to create tech artefacts akin to consent artefacts for establishing liability chains is problematic on multiple fronts:
No jurisdiction has achieved such standardisation or automation of liability allocation.
Automating or augmenting liability apportionment without clear standards violates principles of natural justice by potentially assigning liability arbitrarily.
The idea of "spreading and distributing liability" among participants oversimplifies complex legal doctrines and undermines rule-of-law principles. Liability cannot be treated as a quantifiable commodity subject to technological augmentation.
This aspect reflects an overreach that fails to consider the legal complexities involved in enforcing liability chains.
Assumptions About Objective Technical Assistance: The proposal for extending "appropriate technical assistance" assumes that the techno-legal approach is inherently objective. However:
The lack of standardisation in techniques undermines this assumption.
Without clear benchmarks or criteria for what constitutes "appropriate" assistance, this aspect becomes self-referential and overly broad.
While there may be merit in leveraging technical assistance for governance purposes, it requires acknowledgment of the need for standardisation before implementation.
Part C: Feedback on Gap Analysis
The need to enable effective compliance and enforcement of existing laws
On deepfakes, the report is accurate to mention that existing laws should be effectively endorsed. In the case of deepfakes, while the justification to adopt technological measures is reasonable, the overreliance on watermarking techniques might not be feasible necessarily:
Watermarking Vulnerabilities: As highlighted in the 2024 Seoul AI Summit Scientific Report, watermarks can be removed or tampered with by malicious actors, rendering them unreliable as a sole mechanism for deepfake detection. This limits their effectiveness in ensuring accountability across the lifecycle of AI-generated content.
Scalability Issues: Implementing immutable identities for all participants in a global AI ecosystem would require unprecedented levels of standardisation and cooperation. Such a system is unlikely to function effectively without robust international agreements, which currently remain fragmented.
Make deepfake detection methods open-source and keep improving upon the human and technical forensic methods to detect AI-generated content, be it multi-modal or not, should be the first step. This approach helps in improving upon the relied methods of content provenance.
Cyber security
The reference to cybersecurity law obligations in the report is accurate. However, the report does not give enough emphasis on cybersecurity measures at all, which is disappointing.
Intellectual property rights
Training models on copyrighted data and liability in case of infringement: The report fails to provide any definitive understanding on copyright aspects, and leaves it to mere questions, which is again disappointing.
AI led bias and discrimination
This wording on 'notion of bias' is commendable for its clarity and precision in addressing the nuanced issue of bias in decision-making. By emphasizing that only biases that are legally or socially prohibited require protection, it avoids the unrealistic expectation of eliminating all forms of bias, which may not always be harmful or relevant.
Part D: Feedback on Recommendations
To implement a whole-of-government approach to AI Governance, MeitY and the Principal Scientific Adviser should establish an empowered mechanism to coordinate AI Governance
The supposed empowered mechanism of a Committee or a Group as proposed must take into consideration that without enough capacity-building measures, having a steering committee-like approach to enable whole-of-government approach will be only solution-obsessed and not helpful. Thus, unless the capacity-building mandate to anticipate, address & understand what limited form of AI governance for near-about important areas of priority, like incident response, liability issues, or accountability, are not dealt with enough settled legal, administrative and policy positions, the Committee / Group would not be productive or helpful enough to promote a whole-of-government approach.
To build evidence on actual risks and to inform harm mitigation, the Technical Secretariat should establish, house, and operate an AI incident database as a repository of problems experienced in the real world that should guide responses to mitigate or avoid repeated bad outcomes.
The approach rightly notes that AI incidents extend beyond cybersecurity issues, encompassing discriminatory outcomes, system failures, and emergent behaviors. However, this broad scope risks making the database unwieldy without a clear taxonomy or prioritisation of incident types.
Learning from the AIID, which encountered challenges in indexing 750+ incidents due to structural ambiguities and epistemic uncertainty, it is crucial to define clear categories and reporting triggers to avoid overwhelming the system with disparate data.
Encouraging voluntary reporting by private entities is a positive step but may face low participation due to concerns about confidentiality and reputational risks. The OECD emphasises that reporting systems must ensure secure handling of sensitive information while minimising transaction costs for contributors.
The proposal could benefit from hybrid reporting models (mandatory for high-risk sectors and voluntary for others), as suggested by the OECD's multi-tiered approach.
The proposal assumes that public sector organizations can effectively report incidents during initial stages. However, as noted in multiple studies, most organisations lack the technical expertise or resources to identify and document AI-specific failures comprehensively.
Capacity-building initiatives for both public and private stakeholders should be prioritised before expecting meaningful contributions to the database.
The proposal correctly identifies the need for an evidence base to inform governance initiatives.
Incorporating automated analysis tools (e.g., taxonomy-based clustering or root cause analysis) could enhance the database's utility for identifying patterns and informing policy decisions.
Conclusion
While the pursuit of becoming a "use case capital" has merit in addressing immediate societal challenges and fostering innovation, it should not overshadow the imperative of developing indigenous AI capabilities.
The Dual Imperative
India must maintain its momentum in developing AI applications while simultaneously building capacity for foundational model training and research.
Immediate societal needs are addressed through practical AI applications
Long-term technological sovereignty is preserved through indigenous development
Research capabilities evolve beyond mere application development
Beyond the Hype
The discourse around AI in India must shift from sensationalism to substantive discussions about research, development, and ethical considerations. As emphasized in the Durgapur AI Principles, this includes:
Promoting evidence-based discussions over speculative claims
Fostering collaboration between academia, industry, and government
Emphasizing responsible innovation that considers societal impact
Building trust-based partnerships both domestically and internationally
The future of AI in India depends not on chasing headlines or following global trends blindly, but on cultivating a robust ecosystem that balances practical applications with fundamental research.
留言